Upcoming Seminars
November 21st, 2024
REGISTER NOW!
Spatially resolving antigen receptors in tissues
Established Speaker: Camilla Engblom, Karolinska University, Sweden
Synthetic co-evolution of neutralizing antibodies with SARS-CoV-2
Early Career Speaker: Roy Ehling, Engimmune, Switzerland
Past Seminars
October 24th, 2024
Analysis of human B cell responses in vaccination, infection and allergy with large multiplexed antigen panels
Established Speaker: Scott Boyd, Stanford University, USA
Tissue determinants of the human T cell receptor repertoire
Early Career Speaker: Suhas Sureshchandra, University of California Irvine, USA
September 26th, 2024
The T cell receptor core qualities, as seen by a language model
Established Speaker: Sol Efroni, Bar Ilan University, Israel
Generative Modeling of AIRRs with SoNNia
Early Career Speaker: Giulio Isacchini, Imprint, USA
April 25th, 2024
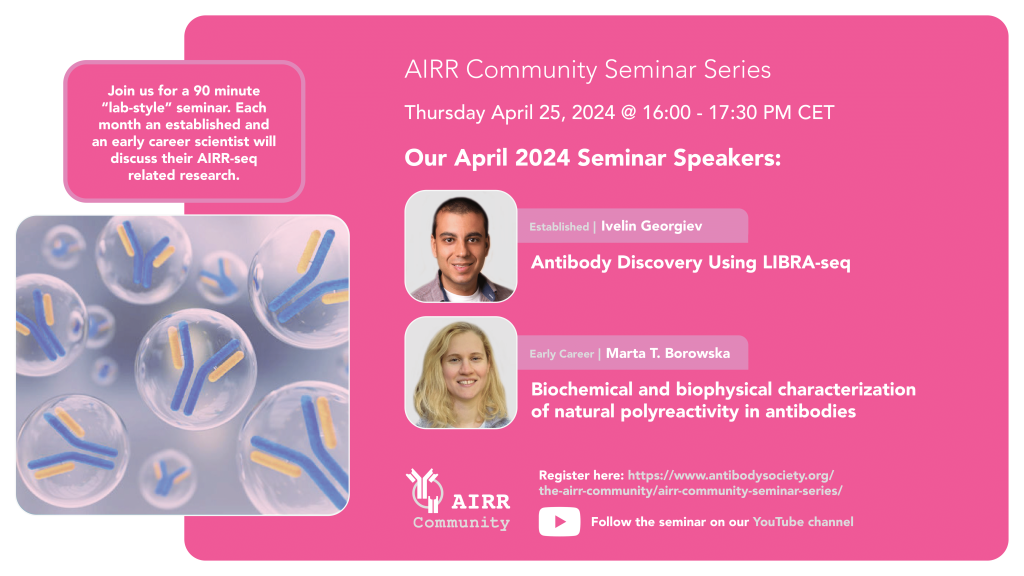
Antibody Discovery Using LIBRA-seq
Established Speaker: Ivelin Georgiev, Vanderbilt University Medical Center
NO RECORDING AVAILABLE FOR THIS TALK
Biochemical and biophysical characterization of natural polyreactivity in antibodies
Early Career Speaker: Marta T. Borowska, Stanford University
March 28th, 2024
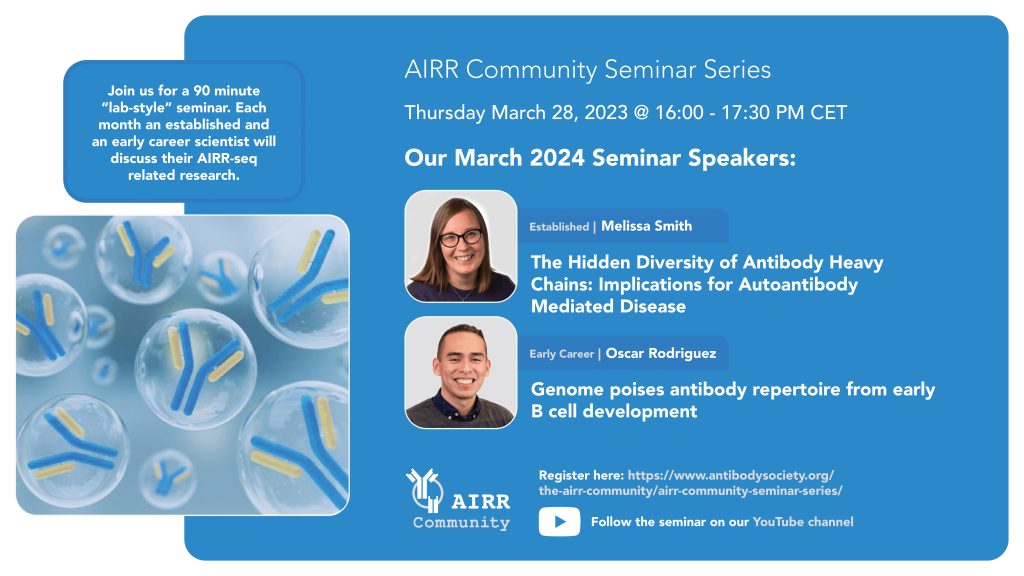
The Hidden Diversity of Antibody Heavy Chains: Implications for Autoantibody Mediated Disease
Established Speaker: Melissa Smith, University of Louisville
Genome poises antibody repertoire from early B cell development
Early Career Speaker: Oscar Rodriguez, University of Louisville
February 22nd, 2024
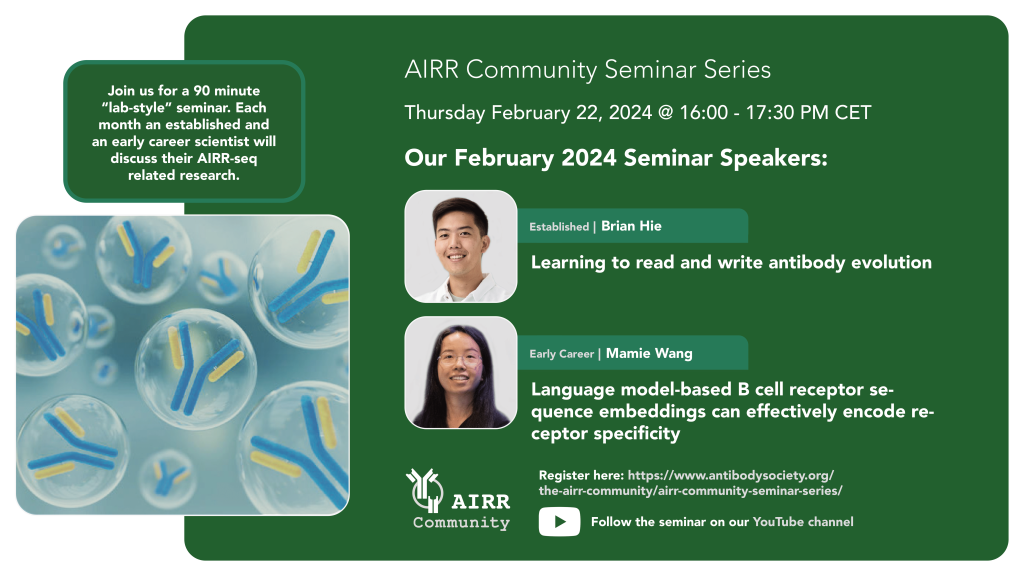
Learning to read and write antibody evolution
Language model-based B cell receptor sequence embeddings can effectively encode receptor specificity
January 25th, 2024
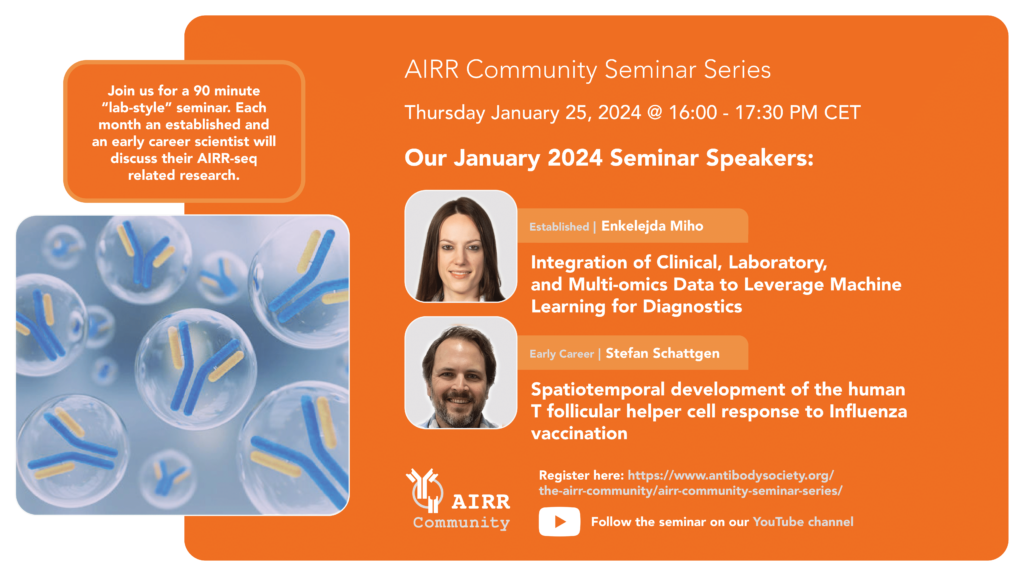
Integration of Clinical, Laboratory, and Multi-omics Data to Leverage Machine Learning for Diagnostics
Established Speaker: Enkelejda Miho, University of Applied Sciences and Arts, Northwestern Switzerland
Talk abstract
Early and accurate disease diagnosis is crucial for preventing disease development and defining therapy strategies. Due to predominantly unspecific symptoms, diagnosis of autoimmune diseases is notoriously challenging. However, multiple types of data are not leveraged for precision diagnostics due to the difficulties of integrating and encoding multi-omics data with clinical and laboratory values, as well as a lack of standardization; clinical decision support systems are often limited to only certain data types. Accordingly, even sophisticated data models fall short when supporting accurate diagnoses and presenting data analyses in a user-friendly form. Therefore, the integration of various data types is not only an opportunity but also a competitive advantage in research and for the industry. We have developed an integration pipeline to enable the use of machine learning for patient classification based on multi-omics data such as genetics, immunomics and metabolomics, in combination with clinical values and laboratory results. Machine learning models resulted in 95% prediction accuracy of autoimmune diseases using integrated data. Our results deliver insights into autoimmune disease research and have the potential to be adapted for applications across disease conditions.
Speaker bio
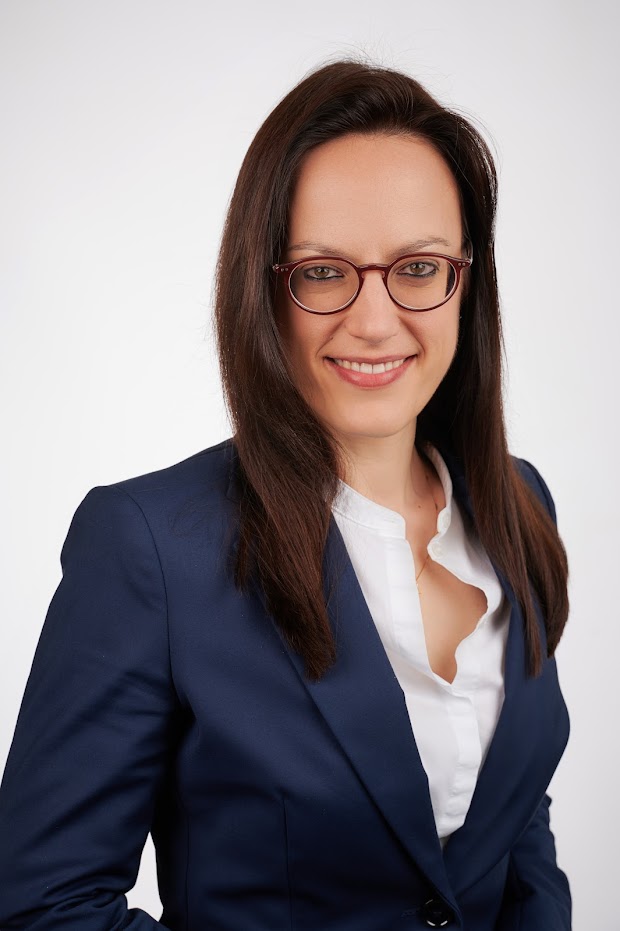
Enkelejda Miho is a professor of Digital Life Sciences and team leader of the Laboratory of Artificial Intelligence in Health (aiHealthLab) as well as group leader at Swiss Bioinformatics Institute. Her research focuses on the use of computer science and artificial intelligence for drug discovery and personalized medicine. The mission of aiHealthLab is to apply artificial intelligence in order to set standards, understand mechanisms and guide decisions in healthcare. The group uses analytics for personalized medicine, drug discovery and development, and support systems in clinics.
Spatiotemporal development of the human T follicular helper cell response to Influenza vaccination
Early Career Speaker: Stefan Schattgen, St. Jude Children’s Research Hospital, USA
Talk abstract
We profiled blood and draining lymph node (LN) samples from human volunteers after influenza vaccination over two years to define evolution in the T follicular helper cell (TFH) response. We show LN TFH cells expanded in a clonal-manner during the first two weeks after vaccination and persisted within the LN for up to six months. LN and circulating TFH (cTFH) clonotypes overlapped but had distinct kinetics. LN TFH cell phenotypes were heterogeneous and mutable, first differentiating into pre-TFH during the month after vaccination before maturing into GC and IL-10+ TFH cells. TFH expansion, upregulation of glucose metabolism, and redifferentiation into GC TFH cells occurred with faster kinetics after re-vaccination in the second year. We identified several influenza-specific TFH clonal lineages, including multiple responses targeting internal influenza proteins, and show each TFH state is attainable within a lineage. This study demonstrates that human TFH cells form a durable and dynamic multi-tissue network.
Speaker bio
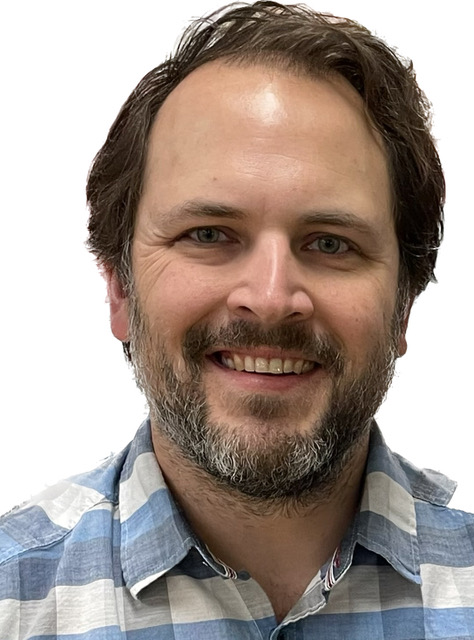
Stefan Schattgen is a research scientist and group leader in the lab of Paul Thomas at St Jude Children’s Research Hospital, USA. In 2015 he received his doctorate in immunology and virology at the University of Massachusetts Medical School where he worked on innate immune sensing of viral infections. He turned his focus towards T cell repertoire biology during his postdoctoral training with Paul Thomas from 2015-2020. His current research interests blend computational and wet lab methods for understanding underlying relationships between a T cell’s specificity and their phenotype and functions during homeostasis, infection, vaccination, and cancer.
November 30th, 2023
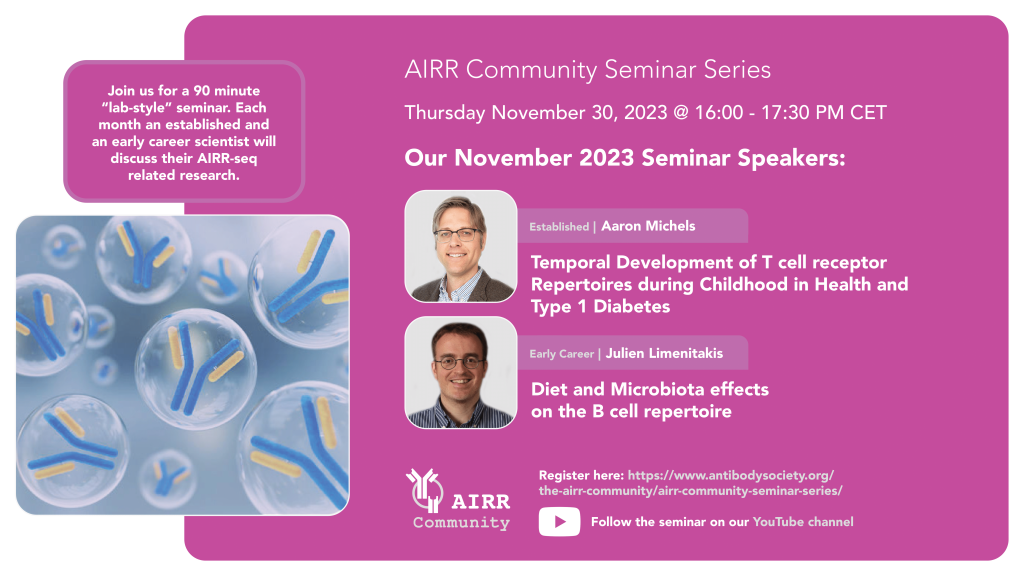
Temporal Development of T cell receptor Repertoires during Childhood in Health and Type 1 Diabetes
Diet and Microbiota effects on the B cell repertoire
Early Career Speaker: Julien Limenitakis, University of Bern, Switzerland
Talk abstract
Colonization by the microbiota causes a marked stimulation of B cells and induction of immunoglobulin, but mammals colonized with many taxa have highly complex and individualized immunoglobulin repertoires. To study this, we opted for a simplified model of defined transient exposures to different microbial taxa in germ-free mice to deconstruct how the microbiota shapes the B cell pool and its functional responsiveness. We previously showed that microbial exposure at the intestinal mucosa generated oligoclonal responses that differed from those in germ-free mice, and from the diverse repertoire that was generated after intravenous systemic exposure to microbiota. Our results reflected a contrast between a flexible response to systemic exposure with the need to avoid fatal sepsis, and a restricted response to mucosal exposure that reflects the generic nature of host–microbial mutualism in the mucosa. A hallmark of mucosal IgA is the high mutational load that accumulates throughout life. This has been mainly interpreted in terms of differences in microbial induction, although once established in early life, the microbiome of humans and experimental mice is relatively stable and mutational activity has been shown to be independent of B cell receptor signaling. Using germ-free and colonized mice provided with different diets formulated with proprietary grain-based processing or from purified chemicals with different principal macronutrient calorie sources, we show that diet affects IgA induction, its repertoire and mutational diversification independently of microbial exposure.
Speaker bio
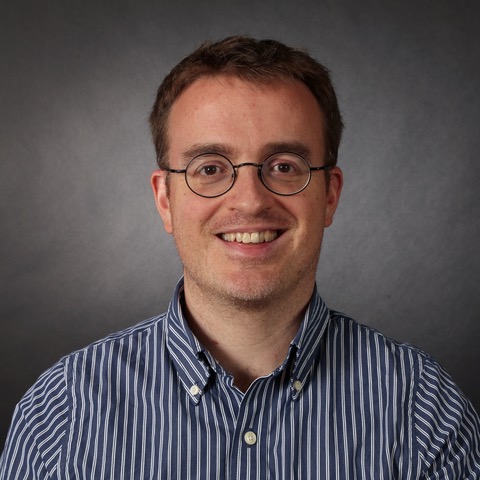
Dr. Julien Limenitakis received his PhD in Microbiology from the University of Geneva. He then joined the Mucosal Immunology group of Andrew Macpherson with a transitional Post-doctoral fellowship from the Swiss Systems Biology Initiative, switching fields to apply systems biology approaches and computational methods to study interactions of gut microbes with the immune system. Currently he is a senior scientist at the University Hospital Bern. His work focuses on how exposure to intestinal microbes, in particular during early life development, shapes B-cell repertoires.
September 28th, 2023
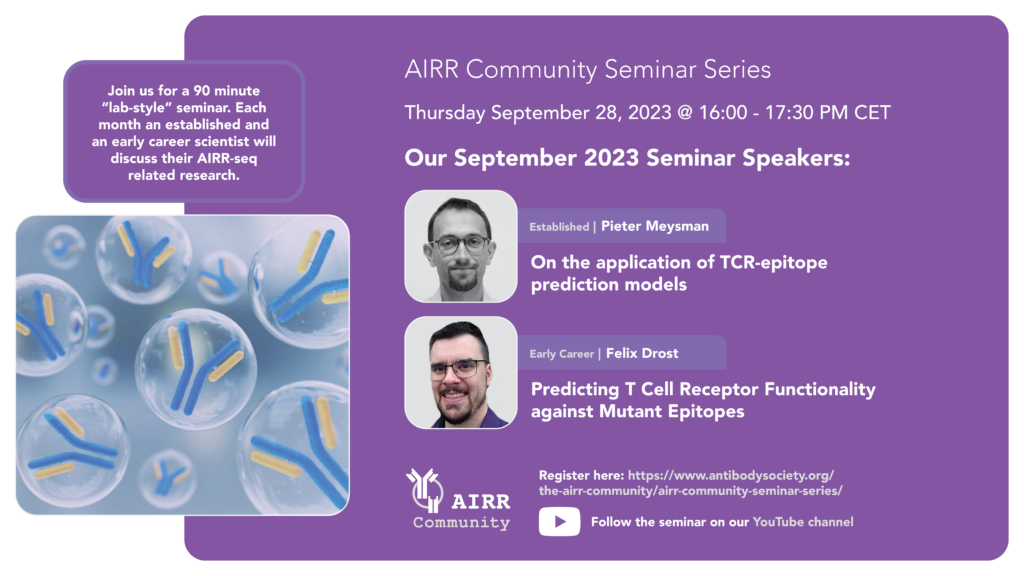
On the application of TCR-epitope prediction models
Established Speaker: Pieter Meysman, University of Antwerp, Belgium
Talk abstract
The recognition of a T-cell epitope target is driven by the unique sequence of the T-cell receptor (TCR). As the TCR sequence theoretically contains all the information that determines its target, it must be possible to infer its target from this sequence. Indeed, within specific settings, we now have performant machine learning models that can address this challenge. It has therefore become necessary to start considering how we can apply these models to gain novel immunological understanding.
In this talk, I want to focus on the application of TCR-epitope prediction models to identify epitope-specific T-cells in full repertoire data, and the additional challenges that are often missed by current evaluation efforts. I also aim to highlight the biggest advancements and milestones that we can expect in the coming years in the field, especially in regard to the unseen epitope prediction problem.
Speaker bio
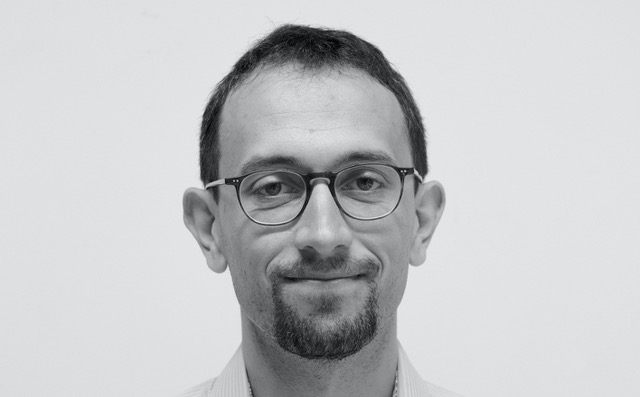
Prof. Pieter Meysman is an associate professor at the University of Antwerp at the ADREM data lab and leads the immunoinformatics activities of the AUDACIS consortium. He graduated as a PhD in bioscience engineering from the KULeuven in 2012, and has published more than 80 research articles and patents. In addition to his academic research, he is part-time CTO of ImmuneWatch, an AI company aiming to decode the T-cell receptor repertoire.
His main research focus is on the use of artificial intelligence to gain understanding into the adaptive immune system. To this end, he has supervised the development of several immunoinformatics tools to link T-cell receptors to their (seen or unseen) targets, including TCRex, ClusTCR and ImRex. He has won a number of awards for his research into the human T-cell receptor repertoire, including most recently the GSK Vaccines award.
Predicting T Cell Receptor Functionality against Mutant Epitopes
Early Career Speaker: Felix Drost, Helmholtz Munich, Germany
Talk abstract
Pathogens and cancer cells can escape recognition by T cell receptors (TCRs) through mutations of immunogenic epitopes. TCR cross-reactivity, i.e., recognition of multiple epitopes with sequence similarities, can counteract such mutational escape, but also may cause severe side effects in cell-based immunotherapies. To predict the effect of epitope mutations on T cell functionality in silico, we present “Predicting T cell Epitope-specific Activation against Mutant versions” (P-TEAM). The Random Forest based model was developed on two comprehensive datasets of murine and human TCRs in response to systematic single-amino acid mutations of their target epitopes to predict T cell reactivity for unobserved mutations, or even unseen TCRs. P-TEAM is complemented with an active learning framework to guide experimental design to minimize primary data acquisition costs. Overall, P-TEAM provides an effective computational tool to study T cell responses against mutated epitopes.
Speaker bio
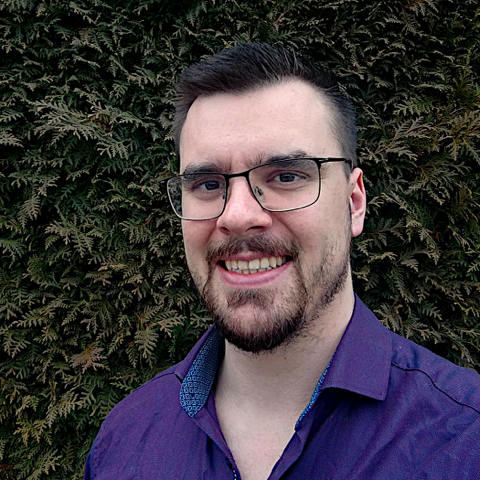
Felix Drost is a doctoral researcher at the Computational Health Center at the Helmholtz Centre Munich under the supervision of Dr. Benjamin Schubert. His goal is to develop effective computational tools for the development of vaccines and biotherapeutics. In his research, he applies machine learning based methods to investigate the T cell Receptor-Epitope landscape through prediction, multimodal integration, and representation learning.
June 29th, 2023
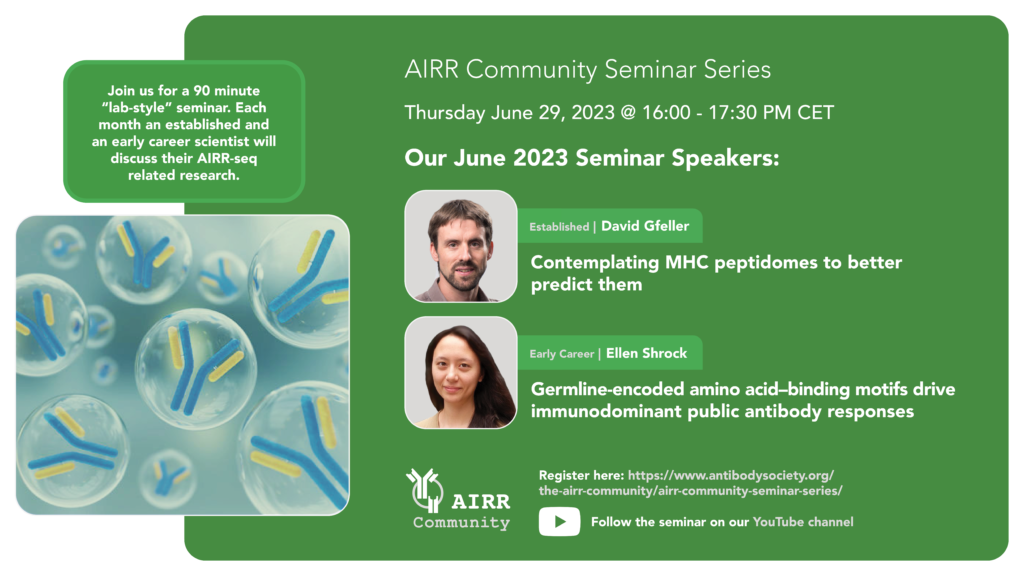
Contemplating MHC peptidomes to better predict them
Established Speaker: David Gfeller, University of Lausanne, Switzerland
Talk abstract
T cells orchestrate the adaptive immune response against pathogens and cancer by recognizing epitopes presented on MHC molecules. The heterogeneity of the MHC peptidome, including the high polymorphism of MHC genes, is influencing TCR repertoires and represents an important challenge towards accurate prediction and identification of T-cell epitopes in different individuals and different species. Here we generated and curated a dataset of more than a million unique MHC-I and MHC-II ligands identified by mass spectrometry. This enabled us to precisely determine the binding motifs of >200 MHC alleles across human, mouse, cattle and chicken. Analysis of these binding specificities combined with X-ray crystallography refined our understanding of the molecular determinants of MHC motifs and revealed alternative binding modes of MHC ligands. We then developed machine learning frameworks to accurately predict binding specificities and ligands of any MHC-I (MixMHCpred) and MHC-II (MixMHC2pred) allele, and further integrated TCR recognition into our epitope prediction pipeline (PRIME). Prospectively applying our tools to SARS-CoV-2 proteins identified several epitopes and TCR sequencing revealed a monoclonal response in effector/memory CD8+ T cells against one of these epitopes with cross-reactivity against the homologous peptides from other coronaviruses. Overall, our work shows how in depth characterization of MHC motifs can help mapping the targets of T cells and understanding TCR cross-reactivity.
Speaker bio
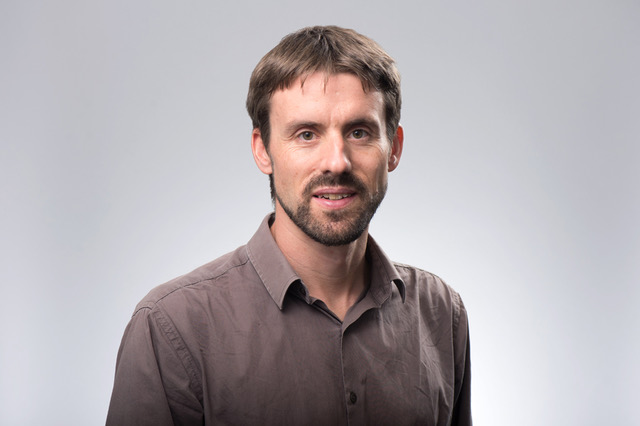
Germline-encoded amino acid–binding motifs drive immunodominant public antibody responses
Early Career Speaker: Ellen Shrock, Harvard University, USA
Talk abstract
Despite the vast diversity of the antibody repertoire, infected individuals often mount antibody responses to precisely the same epitopes within antigens. The immunological mechanisms underpinning this phenomenon remain unknown. By mapping 376 immunodominant “public epitopes” at high resolution and characterizing several of their cognate antibodies, we concluded that germline-encoded sequences in antibodies drive recurrent recognition. Systematic analysis of antibody-antigen structures uncovered 18 human and 21 partially overlapping mouse germline-encoded amino acid–binding (GRAB) motifs within heavy and light V gene segments that in case studies proved critical for public epitope recognition. GRAB motifs represent a fundamental component of the immune system’s architecture that promotes recognition of pathogens and leads to species-specific public antibody responses that can exert selective pressure on pathogens.
Speaker bio
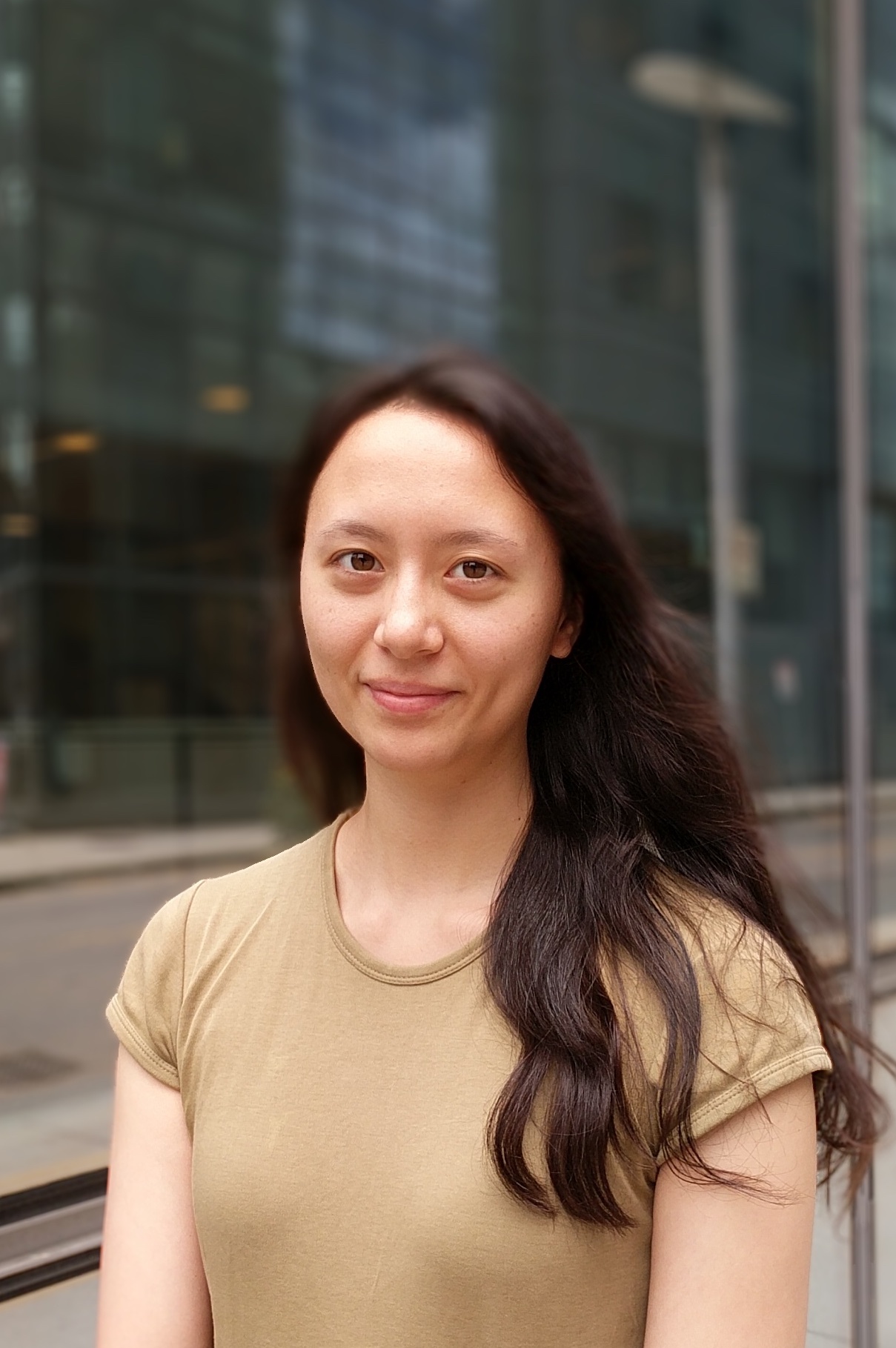
Ellen Shrock received her Ph.D. in Biological and Biomedical Sciences from Harvard University, where she worked in the laboratory of Prof. Stephen Elledge using high-throughput profiling techniques to study the human antibody response to viruses and the mechanisms underlying antigen immunodominance. Ellen received her A.B. in Integrative Biology from Harvard College, where she conducted research with Prof. George Church on genome editing for porcine-to-human xenotransplantation and on the assembly of a synthetic, radically recoded E. coli genome.
May 25th, 2023
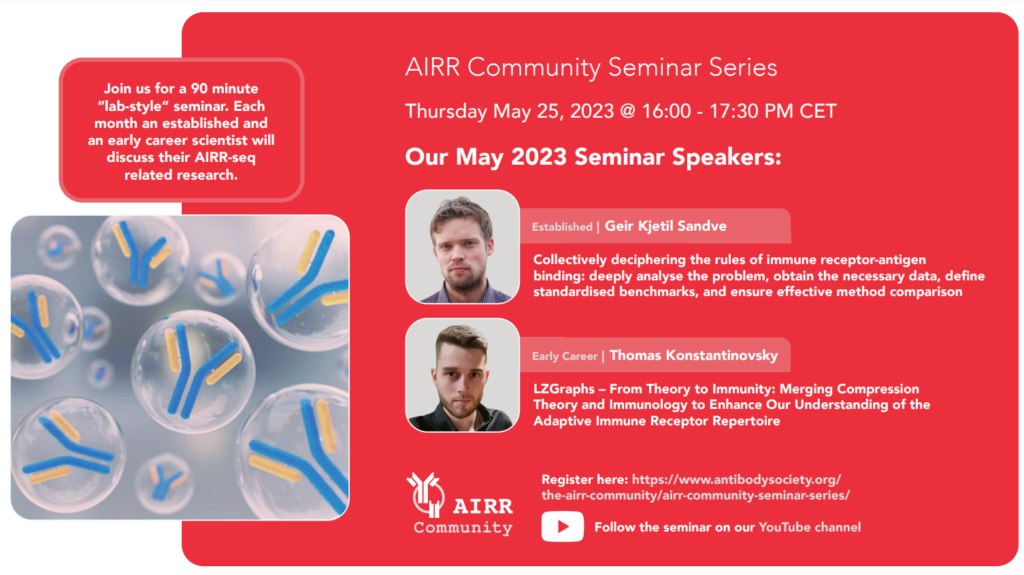
Collectively deciphering the rules of immune receptor-antigen binding: deeply analyse the problem, obtain the necessary data, define standardised benchmarks, and ensure effective method comparison
Established Speaker: Geir Kjetil Sandve, University of Oslo, Norway
Talk abstract
As individual researchers, we are well-trained in designing research projects that address specific and moderately challenging questions. However, when faced with a grand challenge such as understanding antigen recognition by T- and B-cell receptors, how can we, both individually and collectively, effectively tackle this problem? We currently have antigen binding data for only a minuscule proportion of the immune receptor sequence space, and despite the rapid emergence of new machine learning methods, consensus on what constitutes the most promising directions forward remains scarce.
In my talk, I will offer my perspective on this strategic question and discuss some recently published and ongoing work aligned with this strategy. Briefly, I believe the first step should be a rigorous characterisation of the computational challenges of the immune receptor-antigen binding prediction problem. Second, we must ensure that sufficient data is available to guide methodology development, where we in the foreseeable future need to rely on the combined use of experimental and simulated data. Third, we must prioritise interoperability and reproducibility of methods, along with the development of standardised benchmarks, to effectively compare performance and identify limitations of current approaches.
Speaker bio
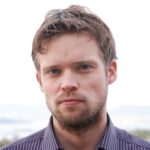
Professor in machine learning at Department of Informatics, University of Oslo. Currently working on deciphering antigen recognition by immune receptors, mainly focused at the sequence level.
LZGraphs – From Theory to Immunity: Merging Compression Theory and Immunology to Enhance Our Understanding of the Adaptive Immune Receptor Repertoire
Early Career Speaker: Thomas Konstantinovsky, Bar Ilan University, Israel
Talk abstract
A new approach that utilizes the Lempel-Ziv 76 algorithm (LZ-76) to encode and represent AIRRs without relying on sequence annotation. The approach involves creating a graph-like model, which enables a wide range of potential applications, including generation probability inference, informative feature vector derivation, sequence generation, sequence analysis, and a new measure for repertoire diversity estimation.
Speaker bio
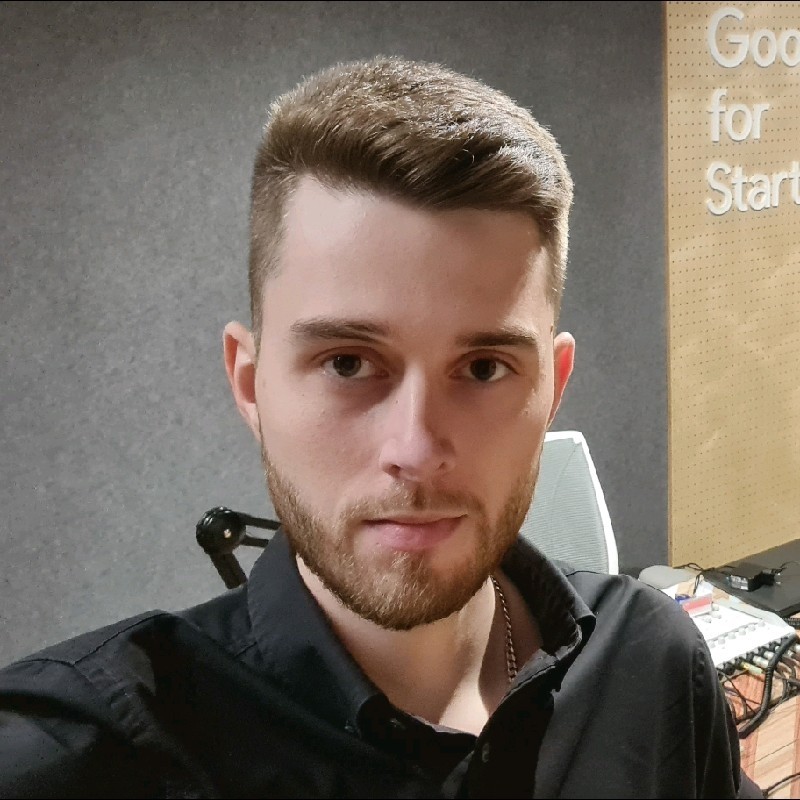
Thomas Konstantinovsky is a computer scientist with a passion for bridging the fields of theoretical and classic computer science with computational immunology. After devoting three years to conducting computational research at the Sagol Research Center of Epigenetics and Aging, He joined Gur Yaari’s lab to pursue his Ph.D. and the goal of developing methods to uncover the mysteries of the adaptive immune system.
April 27th, 2023
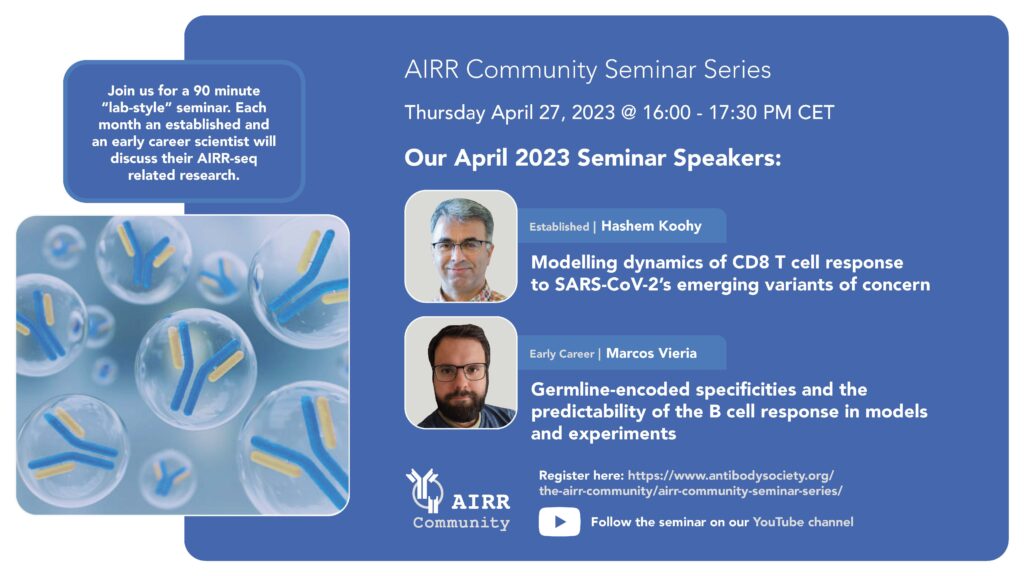
Modelling dynamics of CD8 T cell response to SARS-CoV-2’s emerging variants of concern
Established Speaker: Hashem Koohy, Oxford University, UK
Talk abstract
T cells play a crucial role in our immunity by recognizing and eliminating infected and abnormal cells. T cell response is triggered upon T cell recognition of specific antigens presented by MHC molecules on the surface of target cells. However, the underlying rules of the interactions are incompletely understood. Over the past few years, we have been investigating T cell cross-reactivity and common specificity as two key drivers of T cell antigen specificity. In this seminar, I will focus on T cell cross-reactivity, and will introduce a new deep neural network model that we have developed for accurate and reliable prediction of CD8 T cell targets. I will illustrate how we have been using this model to model the dynamics of CD8 T cell response to emerging SARS-CoV-2 mutant variants.
Speaker bio
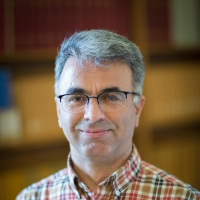
Hashem Koohy is an Associate Professor of Systems Immunology at the MRC Weatherall Institute of Molecular Medicine (MRC WIMM), Oxford University. He leads a research group that aims to understand the basic principles of adaptive immunity using integrative machine learning approaches applied to emerging single-cell sequencing data. His group is specifically interested in decoding the antigen-specific T cell response in time and space.
Hashem was awarded his PhD in Systems Biology from the University of Warwick. He then completed two postdoctoral fellowships at the Sanger and Babraham Institute in Cambridge. In 2017, he moved to Oxford to establish his research group, where he currently leads a research programme.
Germline-encoded specificities and the predictability of the B cell response in models and experiments
Early Career Speaker: Marcos Vieria, University of Chicago, USA
Talk abstract
Antibodies result from the competition of B cell lineages evolving under selection for improved antigen recognition, a process known as affinity maturation. High-affinity antibodies to pathogens such as HIV, influenza, and SARS-CoV-2 are frequently reported to arise from B cells whose receptors are encoded by particular immunoglobulin genes. This raises the possibility that the presence of particular germline genes in the B cell repertoire is a major determinant of the quality of the antibody response. Alternatively, initial differences in germline genes’ propensities to form high-affinity receptors might be overcome by chance events during affinity maturation. We first show how this can happen in simulations: even when fitness differences between germline genes lead to similar gene usage across individuals early on, gene usage can become increasingly dissimilar over time. We next find that mice experimentally infected with influenza virus demonstrate the same pattern of divergence in the weeks following infection. We investigated whether affinity maturation might nonetheless strongly select for particular amino acid motifs across diverse genetic backgrounds, but we found no evidence of convergence to similar CDR3 sequences or amino acid substitutions. These results suggest germline-encoded specificities might enable fast recognition of specific antigens early in the response, but diverse evolutionary routes to high affinity limit the genetic predictability of responses to infection and vaccination in the long term.
Speaker bio
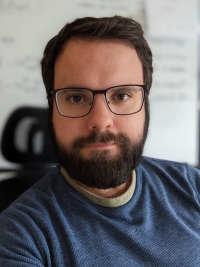
Marcos Vieira is a Senior Research Scientist in the Cobey Lab at the University of Chicago, where he also obtained his PhD. He uses computational, statistical and mathematical tools to study immunity at different scales, from the within-host evolution of B cells to the effects of host immune history on the epidemiology and evolution of viruses.